K-Nearest Neighbors
K-Nearest Neighbors (KNN) is a non-parametric algorithm used for classification and regression. It works by identifying the ‘k’ nearest data points to a given input and then determining the majority class (for classification) or averaging the values (for regression) of these closest points. KNN does not assume a specific form for the data distribution, making it flexible but potentially sensitive to the choice of ‘k’ and the distance metric used.
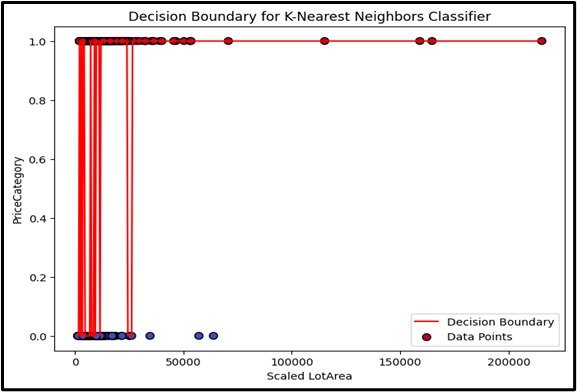
- Concept:K-Nearest Neighbors (KNN) is a non-parametric algorithm used for both classification and regression tasks.
- Non-Parametric:
KNN does not make any assumptions about the underlying data distribution, allowing it to adapt to various patterns. - Distance-Based:
It relies on a distance metric (e.g., Euclidean distance) to identify the nearest neighbors. - Applications:KNN is widely used in:
- Recommendation Systems: Suggesting products based on similar user preferences.
- Image Recognition: Classifying images based on similarity to known examples.

Enhancing Model
Purpose: This is a algorithm classifies data points based on similarity to neighbors.
Input Data:Numerical and categorical variables.
Output: To the lass label or continuous value.

Assumptions
Assumes that similar points are near to each other in the feature space.

Use Case
K-Nearest Neighbors is a good option to choose when you need a simple, instance-based learning algorithm. For example, classifying the species of iris flowers based on sepal and petal length and width.
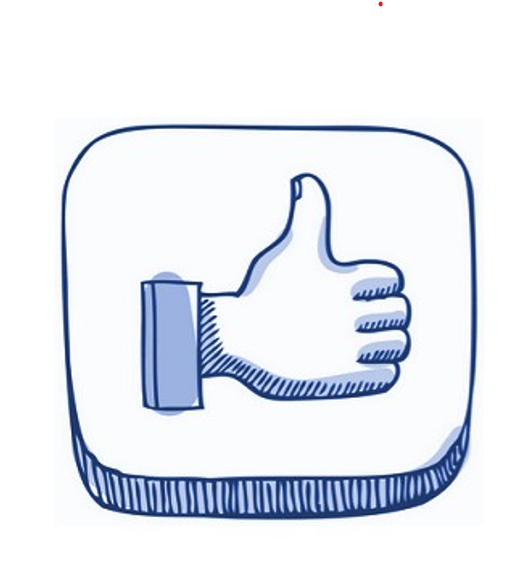
Advantages
- Effective with small to medium-sized datasets.
- No assumptions about the data distribution.
- Adaptable to changes in the data.
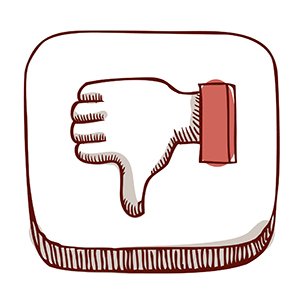
Disadvantages
- It is computationally intensive for large datasets.
- Sensitive to the choice of k and distance metric.
- Requires careful selection of the value of k.
Steps to Implement:
- Import necessary libraries: Use `numpy`, `pandas`, and `sklearn`.
- Load and preprocess data: Load the dataset, handle missing values, and prepare features and target variables.
- Split the data: Use `train_test_split` to divide the data into training and testing sets.
- Import and instantiate KNeighborsClassifier**: From `sklearn.neighbors`, import and create an instance of `KNeighborsClassifier`.
- Train the model: Use the `fit` method on the training data.
- Make predictions: Use the `predict` method on the test data.
- Evaluate the model: Check model performance using evaluation metrics like accuracy, precision, recall, F1 score, or the confusion matrix.
Ready to Explore?
Check Out My GitHub Code